Examining the benefits of using Universal Dynamic Engine (UDE) to manage Covid-19 patient risk and critical treatment load.
When news broke of an obscure respiratory disease emerging from the Wuhan market in early January, few imagined that in just over 2 months the world would be facing the worst pandemic since Spanish flu in 1918. With more than 12M people taking commercial flights every day, Covid-19 has spread around our hyper-mobile world with an unprecedented combination of speed and virulence. Further, without widespread testing for Covid-19, it is difficult to know how the pandemic is spreading and how to appropriately respond to it. While it is certain that the total number of Covid-19 cases is higher than the number of known confirmed cases, the total number of Covid-19 cases is not known.
As the Coronavirus spreads, many are predicting that the demand for beds, ventilators and other treatment resources in hospital will far exceed capacity for Covid-19 patients in the near future. Still, public policy and health community responses to Covid-19 are complex decisions that require a delicate balance between protecting health, the economy, and people’s well-being and emotional health. In times of crisis, reliance on theoretical models present challenges for decision makers, because they don’t always replicate real life well—especially when the virus that causes the pandemic is novel.
Covid-19 Modeling: Dynamics Shouldn’t be Ignored
Healthcare systems are not closed loop, linear systems. Therefore, they cannot be accurately modeled without accounting for various elements (components) of the system which continually influence one another (directly or indirectly) to maintain the system in line with the goals of the system. In the case of a pandemic, these goals include slowing the spread of the disease and providing the highest quality of care possible for those infected.
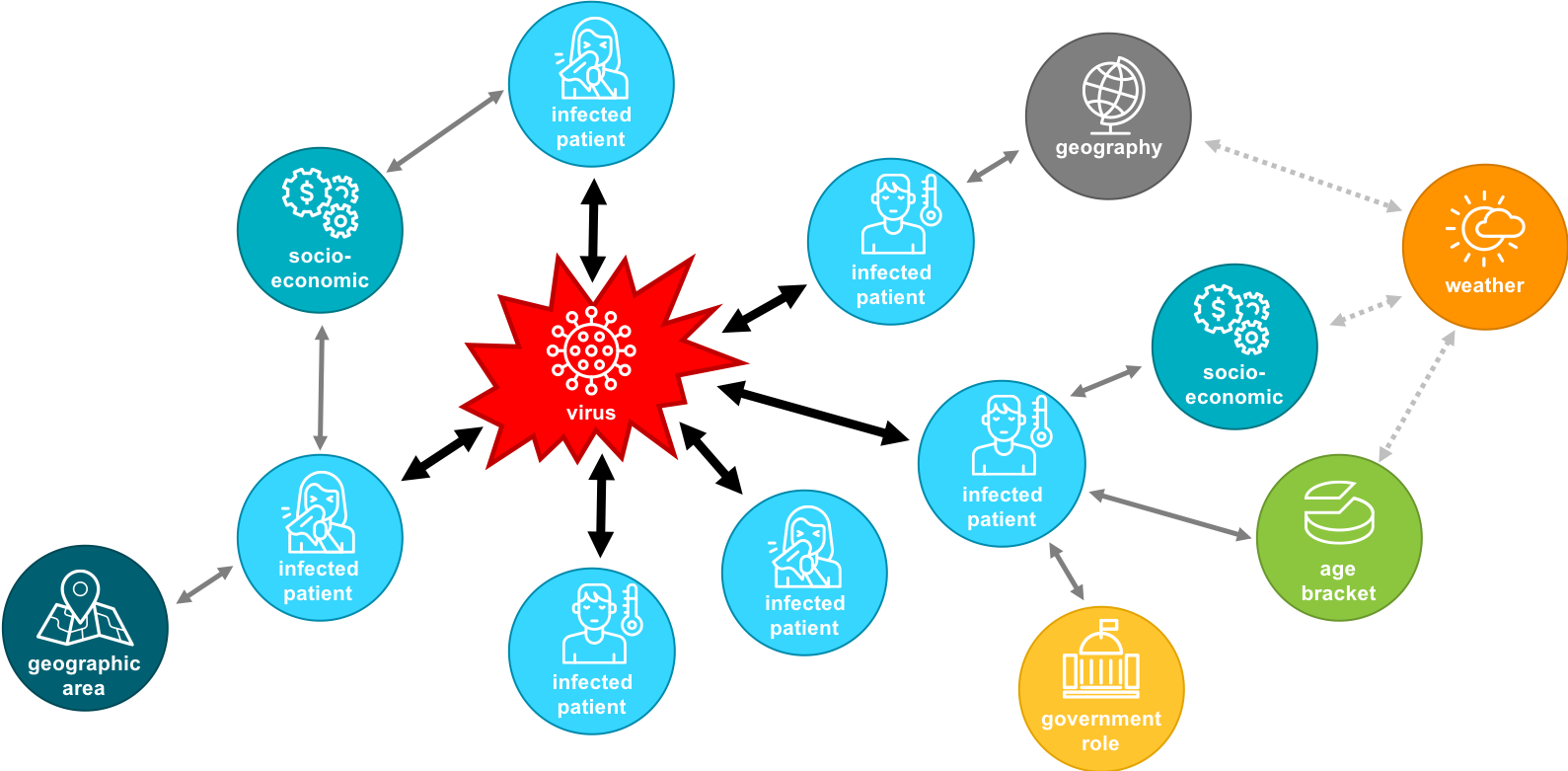
If the relationships between various components of a dynamic system are not well understood, changes made to one part of the chain may affect another in unwanted ways. For instance, treating Covid-19 patients without understanding the influence of pre-existing conditions may place more strain on already overwhelmed medical personnel and hospital resources. A universal mathematical formulation is needed to accurately represent all system dynamics in all cases to provide healthcare system participants with a more complete view of reality. This allows stakeholders to make decisions with more confidence in the outcomes than would be possible if using mechanistic models that may provide a wide range of possibilities and miss new risks due to their dependence on historical data.
UDE and NARS Modeling Capabilities
Universal Dynamics Management (UDE) provides a rigorous process that computes the top-down communicating graphs to deal with the direct and indirect, convergent or degenerative solutions necessary to accurately model the complex, adaptive dynamics of non-linear, open systems. Based on the NARS method of modeling, UDE allows users to accurately represent a high level of interdependent components organized in hierarchy of graphs. Scenario analysis helps modelers construct the remedy and mitigation actions, certify the solution pertinence and discover additional variables and interrelationships that can be dependably used to predict the occurrence of previously known as well as unknown risk behaviors.
Through the use of deconstruction theory, it becomes clear that the overall performance of a system (defined in terms of quantity, quality and cost) does not always represent the expected output of the system given the accumulated characteristics of the constituent components, generally due to a loss in energy. Causal deconstruction allows modelers to uncover results that often defy the common wisdom that stops at the wrong level of analysis and usually produces a host of misleading conclusions.
URM Forum Covid-19 Research
URM Forum has published a discussion paper entitled, “Covid-19: Worldwide Viral Infection Model” as part of our ongoing effort to help government, healthcare and business leaders build the UDE capabilities necessary to accurately represent all system dynamics in all cases. The paper includes results for the digital twin of Covid-19 Crisis Management in Germany, referred to as the CV-19 model, to show how UDE can be applied to help provide critical decision insights as needed to guide actions for any country’s or geographic region’s response to the current pandemic as well as monitor and plan activities in order to avoid a future crisis.
The aim of the presented CV-19 model is to show healthcare system participants how they might use UDE to gain a more complete view of reality as needed to make decisions with more confidence in the outcomes. UDE has been successfully applied for a wide range of healthcare, economic and business uses as the preferred way to predictively characterize dynamic systems under specific conditions; identify singularities and metrics, which define the characteristics of the system under different scenarios of increasing volume and structural changes; and validate the applicability of any proposed corrective solutions.
Join Our Efforts to Deliver Improved Risk Modeling Solutions
The dynamics of our mobile and hyperconnected world amplify the risks. Through the participation of a larger number of constituents, the CV-19 model can be expanded to identify thresholds and help support preparedness for a wider variety of parameters and situations as they relate to any dynamic system, which is influenced directly and indirectly by any number of internal and external forces—including travel patterns, weather, government response and/or socio-economics.
If you are interested in joining our efforts to develop scientific-based solutions to society’s biggest risk management challenges, please contact us.
Learn more about our Covid-19 Modeling and Healthcare Decision Support research
Read the “Covid-19: Worldwide Viral Infection Model” paper to learn how UDE is being applied to rovide critical decision insights as needed to guide actions for any country’s or geographic region’s response to the current pandemic as well as monitor and plan activities in order to avoid a future crisis.